Collection | 22 July 2023
Artificial Intelligence in Photonics and Manufacturing
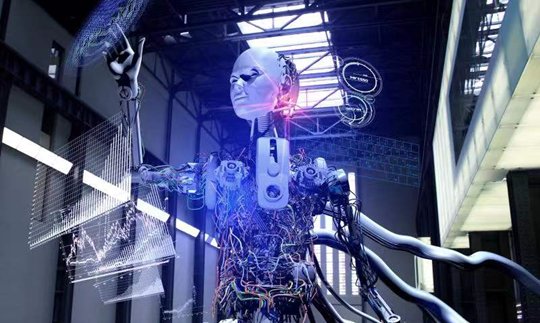
Artificial intelligence is developing dramatically in many fields including photonics and advanced manufacturing. Modern sensing, communication, network, automation and other latest technologies are converged in such applications facilitated by the artificial intelligence. Through the intelligent identification, human-computer/human-machine interaction technology, decision-making and execution technology, as well as cost-effective algorithms, people achieve the intelligence of the design, implementation and manufacturing processes in photonic-related systems. In the last 10 years, the theory of artificial intelligence has been vigorously developed and applied. Novel high-performance sensors have been developed rapidly. The traditional automatic control theory is moving towards the direction of intelligence and human-machine integration. The precision of mechanical design and manufacture is increasing. The effectiveness of novel functionalities is explored. These advances have made the theory of photonics related advanced manufacturing evolving and verified in practice, which has formed a huge impact in the fields of advanced instruments and equipment, smart factories, future optical networking and so on.
This special issue aims to discuss the cutting-edge advances in the field of artificial intelligence in photonics and advanced manufacturing, including the innovative research results and potential applications of artificial intelligence theories and methods, design and application of novel photonic devices/sensors, innovative automatic control technology, human-computer interaction and fusion, smart factories, and the advanced functionalities in photonic systems/networks. We hope to provide an overview as well as insightful perspectives of the field by collecting contributions from leading experts and major industry players to promote scientific breakthroughs and impactful applications in photonics and advanced manufacturing.
This issue is co-edited by
Prof. David Brady, University of Arizona, USA
Prof. Hui Qiao, Tsinghua University, China
Prof. Dapeng Tian, Changchun Institute of Optics, Fine Mechanics and Physics, Chinese Academy of Sciences, China
Prof. Lei Tian, Boston University, USA
-
Article
Motion-copying method with symbol sequence-based phase switch control for intelligent optical manufacturing
Published , Published online: 08 April 2024 , doi: 10.37188/lam.2024.012Implementation of robot-based motion control in optical machining demonstrably enhances the machining quality. The introduction of motion-copying method enables learning and replicating manipulation from experienced technicians. Nevertheless, the location uncertainties of objects and frequent switching of manipulated spaces in practical applications impose constraints on their further advancement. To address this issue, a motion-copying system with a symbol-sequence-based phase switch control (SSPSC) scheme was developed by transferring the operating skills and intelligence of technicians to mechanisms. The manipulation process is decomposed, symbolised, rearranged, and reproduced according to the manufacturing characteristics regardless of the change in object location. A force-sensorless adaptive sliding-mode-assisted reaction force observer (ASMARFOB), wherein a novel dual-layer adaptive law was designed for high-performance fine force sensing, was established. The uniformly ultimate boundedness (UUB) of the ASMARFOB is guaranteed based on the Lyapunov stability theory, and the switching stability of the SSPSC was examined. Validation simulations and experiments demonstrated that the proposed method enables better motion reproduction with high consistency and adaptability. The findings of this study can provide effective theoretical and practical guidance for high-precision intelligent optical manufacturing.
-
Article
Quantitative phase imaging (QPI) through random diffusers using a diffractive optical network
Published , Published online: 22 July 2023 , doi: 10.37188/lam.2023.017Quantitative phase imaging (QPI) is a label-free computational imaging technique used in various fields, including biology and medical research. Modern QPI systems typically rely on digital processing using iterative algorithms for phase retrieval and image reconstruction. Here, we report a diffractive optical network trained to convert the phase information of input objects positioned behind random diffusers into intensity variations at the output plane, all-optically performing phase recovery and quantitative imaging of phase objects completely hidden by unknown, random phase diffusers. This QPI diffractive network is composed of successive diffractive layers, axially spanning in total ~70
\begin{document}$ \lambda $\end{document} , where
\begin{document}$ \lambda $\end{document} is the illumination wavelength; unlike existing digital image reconstruction and phase retrieval methods, it forms an all-optical processor that does not require external power beyond the illumination beam to complete its QPI reconstruction at the speed of light propagation. This all-optical diffractive processor can provide a low-power, high frame rate and compact alternative for quantitative imaging of phase objects through random, unknown diffusers and can operate at different parts of the electromagnetic spectrum for various applications in biomedical imaging and sensing. The presented QPI diffractive designs can be integrated onto the active area of standard CCD/CMOS-based image sensors to convert an existing optical microscope into a diffractive QPI microscope, performing phase recovery and image reconstruction on a chip through light diffraction within passive structured layers.
-
Article
Automated optical inspection of FAST’s reflector surface using drones and computer vision
Published , Published online: 05 January 2023 , doi: 10.37188/lam.2023.001The Five-hundred-meter Aperture Spherical radio Telescope (FAST) is the world ’ s largest single-dish radio telescope. Its large reflecting surface achieves unprecedented sensitivity but is prone to damage, such as dents and holes, caused by naturally-occurring falling objects. Hence, the timely and accurate detection of surface defects is crucial for FAST’s stable operation. Conventional manual inspection involves human inspectors climbing up and examining the large surface visually, a time-consuming and potentially unreliable process. To accelerate the inspection process and increase its accuracy, this work makes the first step towards automating the inspection of FAST by integrating deep-learning techniques with drone technology. First, a drone flies over the surface along a predetermined route. Since surface defects significantly vary in scale and show high inter-class similarity, directly applying existing deep detectors to detect defects on the drone imagery is highly prone to missing and misidentifying defects. As a remedy, we introduce cross-fusion, a dedicated plug-in operation for deep detectors that enables the adaptive fusion of multi-level features in a point-wise selective fashion, depending on local defect patterns. Consequently, strong semantics and fine-grained details are dynamically fused at different positions to support the accurate detection of defects of various scales and types. Our AI-powered drone-based automated inspection is time-efficient, reliable, and has good accessibility, which guarantees the long-term and stable operation of FAST.
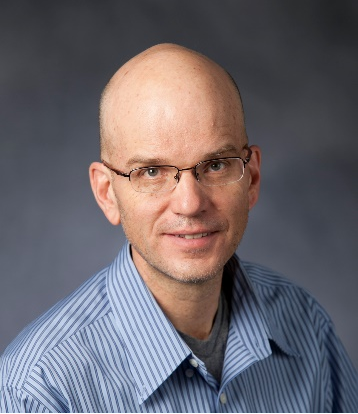
David Brady is the J. W. and H. M. Goodman Professor of Optical Sciences at the University of Arizona. Previously, he was the Fitzpatrick Professor of Photonics at Duke University and founding director of Duke’s Fitzpatrick Institute. Prior to joining Duke, he was on the ECE faculty at the University of Illinois. Brady is a Fellow of SPIE, Optica and IEEE and received the 2013 SPIE Denis Gabor Award for his work on compressive holography. Brady led the team that built the world’s first gigapixel camera and has made significant contributions to x-ray scatter tomography, computational millimeter wave imaging and coded mass spectroscopy. His Ph.D. and early career work pioneered adaptive optical neural networks. He is the author of the text “Optical Imaging and Spectroscopy.”
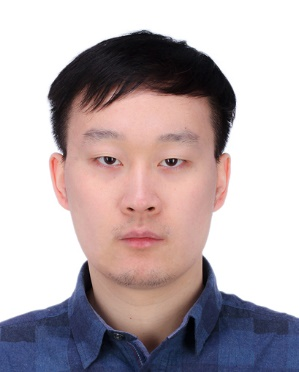
Hui Qiao is currently an Assistant Professor in the Department of Automation at Tsinghua University. From 2019 to 2021, he was a postdoctoral researcher in the Department of Automation at Tsinghua University. Dr. Qiao was selected into National Postdoctoral Program for Innovative Talents in 2019. His research interests include computational imaging, biomedical engineering, and artificial intelligence. He has published papers in the journals such as Cell, Light: Science & Applications, Patterns, IEEE TPAMI and so on.
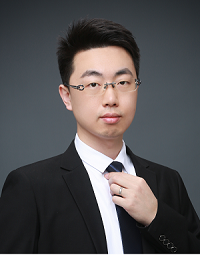
Dapeng Tian is currently a Full Professor with the Key Laboratory of Airborne Optical Imaging and Measurement, Changchun Institute of Optics, Fine Mechanics and Physics, Chinese Academy of Sciences. He is Senior Member of IEEE, CIS, COS, CSOE. He is the author and co-author of more than 80 papers. He holds eighteen Chinese patents, and also won the awards of First Prize of National Science and Technology Progress of China and other high-level science and technology awards. His current research interests include motion control, high accuracy instruments and its manufactory.
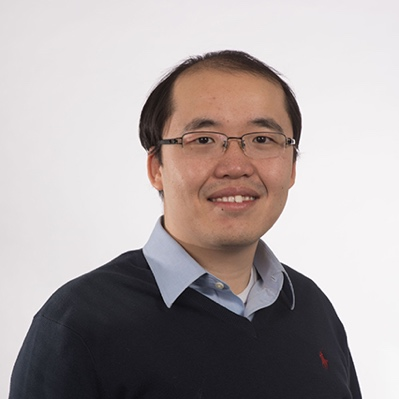
Lei Tian is an Assistant Professor at Boston University. He received his Ph.D. (2013) and M.S. (2010) from MIT. He was a postdoctoral associate at University of California, Berkeley 2013-2016. Dr. Tian’s awards include NSF CAREER award, the 2021 Boston University College of Engineering Early Career Excellence in Research Award, the 2018 Boston University College of Engineering Dean’s Catalyst Award, the 2018 SPIE Fumio Okano Best 3D Paper Prize, the 2014 OSA Imaging Systems and Applications Best Paper Award, and the 2011 OSA Emil Wolf Outstanding Student Paper Prize.